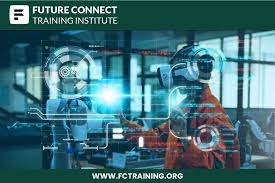
In today’s data-driven world, the ability to analyze and interpret data has become an indispensable skill. Data analysis is not just for data scientists or statisticians; it has permeated various fields, from business and healthcare to social sciences and engineering. A well-structured data analysis course can equip individuals with the knowledge and tools needed to make data-driven decisions, uncover hidden patterns, and predict future trends.
The Importance of Data Analysis
Data is often referred to as the new oil. However, raw data, like crude oil, needs to be refined to extract valuable insights. Data analysis serves as this refining process, transforming raw data into actionable knowledge. Organizations across industries rely on data analysis to optimize operations, enhance customer experiences, and gain a competitive edge. For instance, businesses use data analysis to understand customer behavior, forecast demand, and improve supply chain efficiency. In healthcare, data analysis helps in predicting disease outbreaks, personalizing treatment plans, and improving patient outcomes.
Components of a Comprehensive Data Analysis Course
A robust data analysis course typically covers several key components:
-
Introduction to Data Analysis: This foundational module introduces the basics of data analysis, including different types of data (quantitative vs. qualitative), data collection methods, and the significance of data in various contexts.
-
Statistical Foundations: Understanding statistical principles is crucial for data analysis. This section covers descriptive statistics (mean, median, mode, standard deviation), inferential statistics (hypothesis testing, confidence intervals), and probability theory.
-
Data Cleaning and Preprocessing: Raw data often contains inconsistencies, missing values, and errors. Data cleaning and preprocessing involve techniques to prepare data for analysis, such as handling missing data, removing duplicates, and normalizing data.
-
Exploratory Data Analysis (EDA): EDA is a critical step where analysts use visual and quantitative methods to explore data sets, identify patterns, and formulate hypotheses. Tools such as histograms, scatter plots, and correlation matrices are commonly used in this phase.
-
Data Visualization: Effective data visualization is key to communicating insights clearly. This module covers best practices for creating graphs, charts, and dashboards using tools like Tableau, Power BI, and Matplotlib.
-
Machine Learning and Predictive Analytics: Advanced courses often include machine learning techniques for predictive analytics. Topics may include regression analysis, classification, clustering, and neural networks, using programming languages like Python and R.
-
Practical Applications and Case Studies: Real-world case studies help learners apply theoretical knowledge to practical scenarios. This can include analyzing business data, conducting market research, or evaluating public health data.
-
Ethics and Data Privacy: With the increasing use of data, ethical considerations and data privacy have become paramount. This module addresses ethical issues in data analysis, data protection laws, and best practices for ensuring data privacy.
Benefits of Taking a Data Analysis Course
-
Career Advancement: Proficiency in data analysis is highly sought after by employers. Whether you are looking to enhance your current role or pivot to a new career, data analysis skills can open up a multitude of opportunities in fields such as business analytics, data science, and market research.
-
Improved Decision-Making: Data-driven decision-making leads to better outcomes. By grounding decisions in solid data analysis, individuals and organizations can minimize risks and identify opportunities more effectively.
-
Problem-Solving Skills: Data analysis fosters a systematic approach to problem-solving. Analysts learn to break down complex problems, gather and process relevant data, and derive actionable insights.
-
Increased Efficiency: Data analysis helps streamline processes and improve operational efficiency. By identifying bottlenecks and inefficiencies, organizations can implement data-driven strategies to enhance productivity.
-
Personal Growth: Learning data analysis promotes critical thinking and analytical skills, which are valuable in both professional and personal contexts. It encourages a curious mindset and a methodical approach to tackling challenges.
Conclusion
In conclusion, a data analysis course offers a wealth of knowledge and practical skills that are increasingly relevant in today’s data-centric world. Whether you are a professional looking to upskill, a student preparing for a future career, or simply a curious individual interested in understanding the power of data, mastering data analysis can significantly impact your ability to interpret and leverage data effectively. By investing in a comprehensive data analysis course, you can unlock new opportunities, enhance your problem-solving capabilities, and contribute to informed, data-driven decision-making processes.
4o