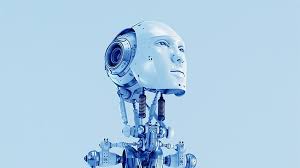
Quality assurance (QA) has always been a critical component of software development, ensuring that products meet specified requirements and are free of defects. Traditionally, QA has been a manual and time-consuming process, involving repetitive tasks and human error. However, the advent of artificial intelligence (AI) is revolutionizing the field, bringing unprecedented efficiency and accuracy. This article explores how AI in quality assurance is changing the game, transforming QA processes, and delivering superior software products.
The Traditional QA Landscape
Traditionally, QA involved manual testing, where testers would execute test cases by hand, record results, and report defects. This process was not only labor-intensive but also prone to human error. Additionally, manual testing could not keep pace with the rapid development cycles of modern software projects, often leading to delayed releases and missed defects.
Automation tools emerged as a solution to these challenges, allowing testers to automate repetitive tasks and execute tests faster. However, traditional automation tools had limitations. They required significant upfront investment in scripting and maintenance, and they could only execute predefined test cases, limiting their ability to identify unexpected issues.
Enter AI in Quality Assurance
AI is transforming QA by introducing intelligent automation, predictive analytics, and advanced data processing capabilities. Here are some key ways AI is revolutionizing quality assurance:
1. Intelligent Test Automation
AI-powered test automation tools can dynamically generate and execute test cases based on user behavior and historical data. Unlike traditional automation tools, which rely on predefined scripts, AI-driven tools can learn from past test results and adapt to changes in the software. This reduces the need for manual intervention and ensures that tests remain relevant and effective even as the software evolves.
For instance, AI can analyze user interaction data to identify the most frequently used features and prioritize testing efforts accordingly. This targeted approach not only improves test coverage but also ensures that critical functionalities are thoroughly tested.
2. Predictive Analytics
AI’s ability to analyze vast amounts of data and identify patterns enables predictive analytics in QA. By analyzing historical defect data, AI can predict where defects are most likely to occur in new software releases. This allows QA teams to focus their efforts on high-risk areas, reducing the likelihood of critical defects slipping through the cracks.
Predictive analytics also helps in estimating the impact of identified defects on the overall system. AI can simulate different scenarios to determine the potential consequences of defects, enabling teams to prioritize fixes based on their severity and impact.
3. Enhanced Test Coverage
AI can enhance test coverage by automatically generating a wide range of test scenarios. By leveraging techniques such as machine learning and natural language processing, AI can understand the application’s functionality and generate test cases that cover different user interactions and edge cases.
For example, AI can analyze application logs and user feedback to identify common usage patterns and generate test cases that replicate these scenarios. This ensures that the software is tested under real-world conditions, improving its reliability and robustness.
4. Improved Defect Detection
AI-powered defect detection tools can analyze code and identify potential defects with high accuracy. These tools use machine learning algorithms to learn from past defects and code changes, enabling them to detect anomalies and patterns that may indicate the presence of defects.
AI can also assist in identifying the root cause of defects by analyzing the relationships between different code components and tracing the flow of data. This speeds up the debugging process and reduces the time required to fix defects.
5. Continuous Testing and Monitoring
AI enables continuous testing and monitoring, allowing QA teams to identify and address issues in real-time. By integrating AI with continuous integration and continuous delivery (CI/CD) pipelines, teams can automatically trigger tests and receive immediate feedback on the quality of the software.
AI-driven monitoring tools can analyze application performance and user behavior in real-time, detecting anomalies and performance bottlenecks. This proactive approach helps in identifying issues before they impact end-users, ensuring a seamless user experience.
6. Enhanced Collaboration and Communication
AI can facilitate better collaboration and communication within QA teams and across different departments. AI-powered tools can automatically generate detailed reports and dashboards, providing real-time insights into the quality of the software. These reports can be shared with stakeholders, enabling informed decision-making and fostering a culture of transparency and accountability.
AI can also assist in managing and prioritizing test cases and defects, ensuring that teams focus on the most critical issues. By automating routine tasks, AI frees up QA professionals to focus on more strategic activities, such as test planning and analysis.
Challenges and Considerations
While AI in quality assurance offers numerous benefits, it also presents some challenges and considerations:
1. Data Quality and Availability
AI relies on large amounts of high-quality data to train its algorithms. Ensuring that data is accurate, complete, and representative of real-world scenarios is crucial for the success of AI in QA. Organizations must invest in data collection, cleaning, and preprocessing to harness the full potential of AI.
2. Skillset and Training
Implementing AI in QA requires a skilled workforce with expertise in AI and machine learning. Organizations must invest in training their QA teams and providing them with the necessary tools and resources to effectively leverage AI. Collaborating with AI experts and data scientists can also accelerate the adoption of AI in QA processes.
3. Ethical and Security Considerations
AI introduces ethical and security considerations, such as data privacy and bias. Organizations must ensure that their AI models are transparent, explainable, and free from bias. Implementing robust security measures to protect sensitive data is also essential to prevent unauthorized access and data breaches.
The Future of AI in Quality Assurance
The future of AI in quality assurance is promising, with advancements in AI technologies expected to drive further innovation. Here are some trends to watch:
1. AI-Driven Autonomous Testing
The next frontier in QA is autonomous testing, where AI takes complete control of the testing process. Autonomous testing tools can independently design, execute, and analyze tests, continuously learning and improving their performance. This will significantly reduce the need for human intervention and enable QA teams to focus on higher-level tasks.
2. AI-Powered Quality Gates
AI can act as a quality gate, automatically assessing the quality of software based on predefined criteria. AI-powered quality gates can analyze code changes, test results, and performance metrics to determine if the software meets the required standards. This ensures that only high-quality software is released to production, reducing the risk of defects and improving user satisfaction.
3. Explainable AI in QA
As AI becomes more integrated into QA processes, the need for explainable AI grows. Explainable AI provides insights into how AI models make decisions, enabling QA teams to understand and trust the results. This transparency is crucial for identifying and addressing any biases or errors in AI models, ensuring that they deliver accurate and reliable results.
Conclusion
AI in quality assurance is revolutionizing the way software is tested and validated. By leveraging AI technologies, QA teams can achieve higher efficiency, accuracy, and test coverage, ultimately delivering superior software products. While there are challenges to overcome, the benefits of AI in QA far outweigh the drawbacks, making it a game-changer in the industry. As AI continues to evolve, its impact on quality assurance will only grow, driving further innovation and transforming the future of software testing.